How basys.ai is Building Trustworthy AI for Better Patient Care
- alaayoussef8
- Jan 27
- 3 min read
Updated: Jan 28
Written BY: Alaa Youssef, PhD
VP of AI Solutions & Moonshot - basys.ai
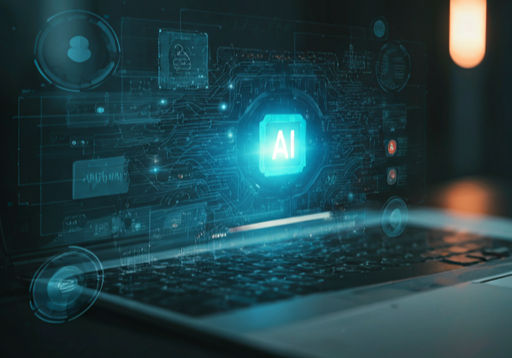
Introduction
Large Language Models (LLMs) have significant potential to transform operational healthcare by analyzing data, supporting clinical decisions, and streamlining workflows. However, generative AI also presents risks, especially in high-stakes environments like healthcare, where the margin for error is non-existent. While frameworks and principles for responsible AI are rapidly evolving, the real challenge lies in translating these principles into actionable know-how—how to effectively apply them in complex, high-stakes settings is the million-dollar question.
The complexity is compounded by the ethical imperative to deploy transformative technologies responsibly. Withholding a technology or treatment that has the potential to benefit society raises moral questions, akin to the "Number Needed to Treat" (NNT) concept in medicine. These considerations highlight the need for robust solutions that balance innovation with safety.
How basys.ai Approaches This Problem
basys.ai takes a comprehensive approach to developing trustworthy AI in healthcare, combining technical innovation, domain expertise, and ethical oversight. By aligning its technology with patient-centered care principles, the company works to improve clinical decision-making and foster trust in AI systems.
The Challenge: Why Guardrails Matter?
Imagine a generative AI system designed to streamline healthcare workflows by summarizing patient histories for clinical decisions. In one case, the AI misrepresented a patient’s medical record, hallucinating critical details such as a history of non-compliance with treatment. Based on this flawed summary, the patient’s prior authorization for a life-saving procedure was denied, delaying care and potentially exacerbating their condition.
The risks of such errors extend beyond immediate harm to the patient. Misinformation in patient summaries undermines clinicians’ trust in AI systems, making them hesitant to rely on these tools in critical decision-making. This lack of trust can hinder the adoption of potentially transformative technologies in healthcare. At the same time, regulatory scrutiny intensifies as healthcare organizations face increased accountability for deploying AI systems that may inadvertently cause harm.
How basys.ai Builds Trustworthy AI
Mission and Ethical Focus
At the heart of basys.ai's mission is a commitment to building AI systems that are not only innovative but also ethical, transparent, and aligned with the core values of patient care. The company believes that the successful integration of AI into healthcare depends on trust, which can only be achieved by addressing risks like hallucinations and biases from the outset.
Developing Robust Guardrails
basys.ai adopts a proactive approach to mitigate risks, incorporating measures to prevent hallucinations and bias at every stage of the AI lifecycle. By emphasizing data quality, human oversight, and continuous monitoring, the company sets a standard for responsible AI development.
Designing the Solution: Building Guardrails
Identifying Vulnerabilities
basys.ai audits its algorithms to uncover potential weaknesses, stress-testing models under diverse conditions to identify where hallucinations or biases might emerge. By simulating real-world scenarios, the company ensures its systems can handle unpredictable inputs.
Data Quality
To combat bias, basys.ai diversifies its training datasets, sourcing data from multiple demographics and clinical settings to ensure representation across age, gender, ethnicity, and geographic regions. Rigorous preprocessing strategies, including thorough labeling and validation, improve data quality and reduce errors.
Human-in-the-Loop Oversight
basys.ai integrates human feedback at critical junctures, ensuring clinicians can validate AI recommendations before action is taken. This collaboration not only prevents errors but also helps refine the system for future use.
Transparency and Explainability
basys.ai prioritizes making AI decisions explainable. Clinicians can trace how recommendations were derived, with clear reasoning tied to specific data points. Explainable AI (XAI) tools foster trust and accountability by providing interpretable evidence for every decision.
Continuous Monitoring and Improvement
The company employs automated feedback loops and real-time monitoring to identify and rectify issues as they arise. Regular audits and updates keep the models accurate, compliant, and effective over time while adapting to evolving clinical practices and regulatory requirements.
Real-World Impact: Accelerating Diabetes Care with AI-Driven Precision
According to the CDC, in 2021, 38.4 million people of all ages—or 11.6% of the U.S. population—had diabetes, highlighting the pressing need for efficient and accurate healthcare solutions. Through its partnership with Mayo Clinic, basys.ai applied its advanced AI platform to diabetes use cases, revolutionizing the prior authorization (PA) process for this widespread condition.
Traditionally, PA required human reviewers to spend 20 to 90 minutes per case navigating complex medical charts, often causing delays that put timely care at risk. basys.ai addressed this challenge by leveraging longitudinal data and integrating robust guardrails into its platform. The system quickly and accurately analyzed patient medical histories and seamlessly matched payer policies with patient-specific attributes, ensuring that medication adjustments were justified and compliant.
As a result, the PA process was completed in minutes instead of hours, allowing patients to access essential medication adjustments promptly. This innovation not only improved operational efficiency but also ensured timely and appropriate care, demonstrating how basys.ai’s AI-driven solutions are effectively addressing inefficiencies in the healthcare system while improving outcomes for millions of patients living with diabetes.
Comentarios